How to Mitigate Shadow AI Security Risks by Implementing the Right Policies
Security has become even more paramount in every organization with growing popularity in AI created by a new phenomenon of ‘Shadow AI’ applications that need to be vetted against security policies.
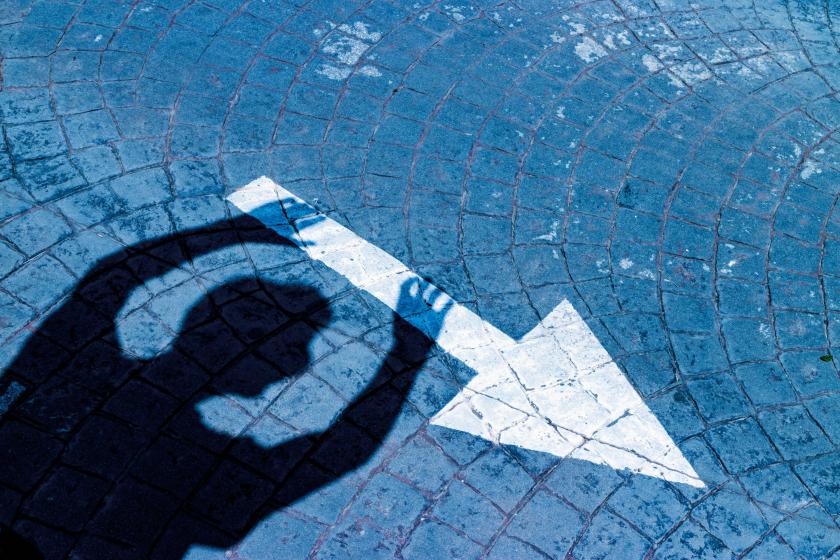
The ‘Shadow IT’ of several years ago is now evolving to ‘Shadow AI.’ The growing popularity of LLM or Multi-modal Language Models has led to product teams within an organization adopting these models to build productivity-enhancing use cases. There has been an explosion of tools and cloud services simplifying the build and deployment of GenAI applications by teams in marketing, sales, development, legal, HR, etc. While there is rapid adoption of GenAI, security teams are yet unclear on the implications and policies. Product teams who are building applications are not waiting for the security teams to catch up, thereby causing potential security concerns.
IT and security teams are contending with unauthorized applications that can lead to threats of network infiltration, data breach, and disruption. At the same time, organizations must avoid an approach that is too heavy-handed, which can stifle innovation and prevent the development of a breakthrough product. Enforcing policies that will prevent users from experimenting with GenAI applications will create a negative impact on productivity and lead to further silos.
The Challenge of Shadow AI Visibility
Shadow IT created a community of employees who used unauthorized devices to support their workloads. It also spawned ‘citizen developers’ who could use no code or low code tools to build applications, without having to go through formal request channels for new software. Today we have Shadow AI citizen developers using AI to build AI applications or other types of software.
These AI-developed applications support productivity and speed up project completion or see how far LLM (Large Language Models) can go in solving a tricky DevOps task. Although not usually malicious, the Shadow AI applications can eat up cloud storage, add to storage costs, introduce threats to the network, and cause a data breach.
How can IT gain visibility into Shadow AI? It’s wise to reinforce the practices set in place to mitigate Shadow IT risks with the caveat that AI’s large language models can make anyone a citizen developer. Thus, the scope of applications and data generated has increased significantly. That means a more complex data protection job for IT teams who must observe, monitor, learn, and then act.
Data Protection in the Shadow AI World
Shadow AI’s output needs to be discovered, analyzed and subject to the same security policies governing other data workloads in the enterprise. Making sure data discovery, monitoring and policy rules enforcement tools are working at peak performance is a vital first step. Analytics can use AI-powered automation tools, working 24x7 to flag unusual behavior and help prevent data privacy and compliance violations.
AI output also requires innovative approaches due to the sheer volume of data being processed and generated, data which, uncontrolled, can cause an organization to be at risk for data privacy regulations. ‘Confidential computing’ is one approach that some companies are engaged in. Basically, it encrypts data while it is processed, so sensitive and private data is not subject to exposure. This is a means of ensuring that the data being used and/or generated by Shadow AI applications will not be subject to risk. The underlying premise here is that organizations realize the flow of data will not be stopped by AI or Shadow AI, and the best approach is risk mitigation via data containment.
Remote Shadow AI Adds Complexity
Current market statistics suggest that remote work is expected to persist as a viable option well beyond the present year. Various research projections indicate that a substantial portion of the workforce from the IT industry, especially those skilled in application development and AI, is leading the trend. Other fields like medical, healthcare, accounting, finance, and marketing also see a significant presence in remote work. All have the opportunity to become Shadow AI practitioners since generative AI is so easily accessible.
Organizations must diligently undertake and proactively apply various remote application security measures in order to help IT better control shadow applications that are unauthorized and not fully vetted. Remote application solutions, for example, can help customers who are already in cloud transformation deploy Zero Trust Architecture (ZTA). This is accomplished by introducing a remote browser isolation solution that evaluates requests against company access policy and security measures. It enables IT to start enforcing ZTA at the cloud level for all users, irrespective of where that user is located or distributed across a global workforce. Another advantage is that it does not require expensive edge equipment.
Four Practices to Prevent Shadow AI Data Leaks
One of the big concerns for organizations in a GenAI world is data security. When entering confidential company information, source code or financial data into AI tools there is a concern about sensitivity of the data and if information is being used to train the foundation models. Certain industries like healthcare or financial services will be more sensitive to these data leakages.
To prevent these types of data leaks, going forward, four practices are needed:
1.) Reinforce all basic security measures governing data flow.
2.) Add AI-specific measures to strengthen security.
3.) Enhance solutions serving remote workers to ensure Shadow AI risk mitigation.
4.) Understand that employees are driven to productivity, and AI can be a benefit when properly controlled.
These practices will ensure a secure data environment.
Kamal Srinivasan is the Senior Vice President of Product and Program Management at Parallels, a part of Alludo.
Related articles:
About the Author
You May Also Like