Enterprise Risk Management: Illuminate the Unknown
Taking risk is how businesses grow; managing risk is how they sustain that growth -- especially under pressure from regulators. Here's how to assemble a risk management architecture that anticipates
December 1, 2005
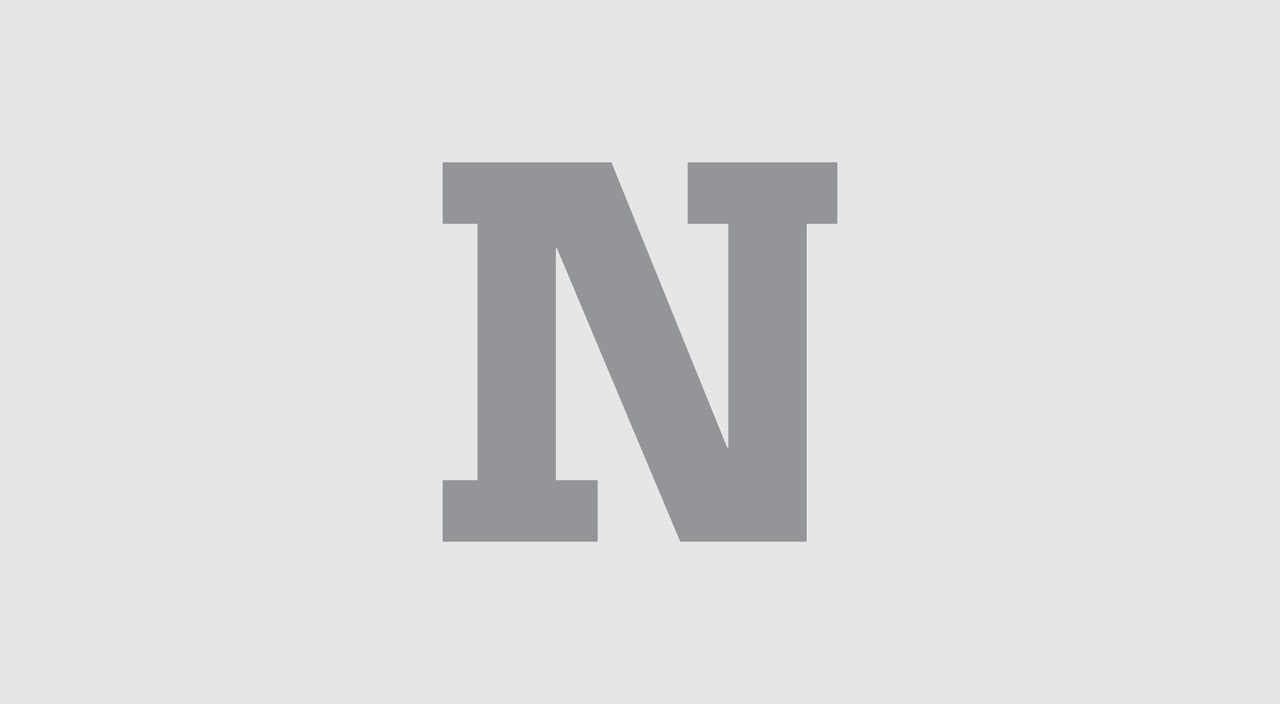
The word "risk" comes from the same root as the Italian verb riscare, which means "to dare." In the quest for competitive advantage, businesses are nothing if not daring. Taking risks is essential. The more an organization can understand, predict and manage the dangers lurking in its path, the more it can turn daring behavior into the stuff of sustained success.
Beyond the insurance industry, the goal of most risk management efforts today is to control the variability of financial outcomes, such as profits and stock prices, while letting corporations pursue increasing levels of profitability and returns. In the financial services industry, intense interest in risk management began two decades ago with the devastating failures of Barings Bank and Long Term Capital Management. Interest has accelerated with the dot-com bubble burst and Enron and WorldCom fiascos. In each case, a major culprit was the lack of organizational control and transparency — in short, a risk management failure.
This article describes how to put together an enterprise risk management (ERM) strategy that factors in all the discrete parts of the problem. With regulatory compliance and increasingly sophisticated threats to business, organizations need the big picture that an architectural approach provides.
ERM: Get the Big Picture
There are many kinds of risk, and, as you'd expect, a lot of "silo-ization" when it comes to managing processes involved. "We had accounting granting access codes to people to look at these transactions, and then we had purchasing coming in and saying 'yep, this person can look at those transactions,'" says Jayne Gibbon, manager of Internal Audit, about the way things used to be at Kimberly-Clark Corp. "Nobody was looking at whether giving the same person access to both kinds of transaction codes would expose the company to fraud."Gibbon worked with a tool suite from Virsa to execute rules and automate processes inside the company's huge SAP R/3 applications to oversee "the trillions of possible combinations" of access codes for authorization. "Virsa can tell us if the risk is too great to approve the authorization combination for particular employees," Gibbon says. "Business, not IT, is ultimately responsible for reducing risk by making sure access is controlled properly. We have to evaluate risk at an enterprise level."
Gaining a global view of risk management is essential for both business leaders and IT, which must look broadly, beyond the silos, to understand all types of risk and what they mean to information flow. Business decision-making must address risk and be supported by business intelligence and data warehousing.
Risk Types and Processes
ERM addresses many kinds of risk, but there are three prevalent types. Market risk (including liquidity risk) is about loss caused by adverse changes in market factors, such as stock prices and interest rates. Credit risk applies to loss due to the inability of counterparties to honor their financial obligations. And operational risk is about failures in operating processes and systems, including security loss and fraud. Also important to consider are legal and regulatory risks, plus the potential of damage to business reputation.
Companies must define their risks precisely. Obviously, businesses actively seek certain kinds of risk. A hedge fund might take on specific kinds of market risk to achieve high returns; payroll processors might provide value through operational risk transference. An example of a detrimental risk might be a danger to manufacturing operations. This is what organizations must reduce or eliminate.
You can categorize risk processes in many ways. The classification system advanced by the Committee of Sponsoring Organizations (COSO) of the Treadway Commission, which is influential in defining causes of fraudulent financial reporting, is a good place to start. As shown in the diagram (right), COSO classifies eight areas for risk management processes:
Internal environment, or the organizational culture that is the foundation for risk management
Objective setting, where the focus is on goals that may be adversely affected by risky events
Identification of risk events that can affect the organization's objectives
Risk assessment, considering the likelihood of a risk event and what its effects would be on organizational goals
Risk response, where the focus is on determining options and assessing the choices for risk mitigation
Control activities, focusing on the policies and procedures to ensure proper execution of the risk response
Information and communication, which is about keeping interested parties (including management, shareholders and regulators) informed
Monitoring, which is about watching over the organization's risk in its management processes.
These eight areas would be prioritized and addressed iteratively depending on where your organization's structure is most scrutinized. The first three areas are clearly process and document driven; technology comes into play mostly in areas four through eight.
The diagram maps these areas against the common risk types. Investment banks, for example, generally have taken on market risk first and then created frameworks incorporating the eight COSO areas. As they've grown to rely on their market risk management, these firms have moved on to credit and operational risk. (Note: The third dimension of the cube reminds us that we must factor in the distinct views that lines of business need to customize risk types and management processes.)
The Enterprise Architecture
Before detailing ERM itself, we need to define the key elements of enterprise architecture. The Open Group Architectural Framework (TOGAF) has become a starting point for many organizations. TOGAF describes all aspects of an organization; here we are primarily interested in the information systems architecture, which is about applications and data, but we can't forget that this architecture must support business processes.A TOGAF enterprise architecture contains six main components:
Transactional systems and data repositories support core business processes. These systems detail transactional information necessary for successful business operations.
Enterprise data repositories aggregate information from large cross-sections of the enterprise. ERM depends on such a view.
Decision-support environments focus on analyzing data in enterprise data repositories.
Business process management (BPM) automation improves organizational efficiency by systematically detecting and proactively responding to relevant events. BPM is a critical area for ERM because risks can rapidly escalate through processes to cause severe losses.
Enterprise data transport depends on data extract, transformation and load (ETL) middleware and service-oriented architecture (SOA) components, such as Web services; it delivers disaggregated information from operational systems to enterprise data repositories and user applications.
Interfaces for corporate stakeholders give user access to components within the enterprise. For risk management and compliance, some important constituents are regulatory authorities, corporate management and investors. These are stakeholders who sit outside mainstream enterprise operations. Applications built to satisfy their demands tap into operational data repositories as well as decision-support environments.
All Together Now: An ERM Framework
The next step is to map risk management components into an enterprise architecture framework. In this section, I will talk about some of enterprise architecture's technology characteristics and requirements as they pertain to risk management and analysis.
Transactional systems and services dominate most enterprises. They record business activity and thus require frequent additions and updates. A good example would be on a securities trading floor, where you'd find some kind of fixed-income derivative trading system used not only for booking trades but also for pricing potential trades, an operation that requires significant computation.
Referring back to our COSO components, transactional services are mostly used in risk assessment and control activities. The most effective way to mitigate risk is to measure and limit potential risk exposures —that is, before transactions reach the enterprise. Some risk assessment techniques, especially those for complex risks, may involve significant amount of computation. In recent years, Value at Risk (VaR) has become popular. A statistical measure that indicates the maximum loss that can be experienced for a given level of confidence, VaR often requires highly sophisticated techniques such as Monte Carlo simulation.
If we consider the trading floor example again, risk management authorities would have to monitor and limit both the credit and market risks that an individual trader could take. Such an assessment would have to happen with blinding speed based on intensive computations to measure and control the potential trade risk.The large number of users and volume of simultaneous activity involved in this scenario puts heavy demands on system scalability and ease of maintenance. Thin-client, application-server architectures have become popular. The compute-intensive nature of this sort of risk assessment and control is a reason why financial services firms are at the leading edge of grid computing and other paradigms that promise increasing power at an affordable price. Risk assessment automation is also opening the door to extending the systems for customer self-service.
Decision-support environments support the assessment, response, information, communication and monitoring components of risk management. Analyzing risk exposure likely involves querying a lot of data in an unpredictable manner; risks must be analyzed both individually and as a group (to study portfolio effects, for example). Although response-time demands are less onerous than with transaction systems, risk modeling also involves significant computation.
Analysts are sophisticated in their use of data, often examining data access patterns across heterogeneous sources. This usually calls for a data warehouse supported by BI tools with functionality for canned "burst" reporting, ad hoc querying and OLAP analysis. Data mining and neural network-based optimizers frequently add to the load carried by decision-support systems.
Corporate stakeholder services meet the requirements of the board, senior management, operations, internal audit groups, regulatory authorities and investors. These users typically need risk management information ranging from tactical control and monitoring reporting to dashboard-based strategic reporting. They access systems in unpredictable ways: drilling down from high-level summaries to relevant granular detail, for example. Of paramount importance is the data's quality and currency.
To be responsive, systems serving corporate stakeholders must be built rapidly and reconfigured easily, often by users and without the aid of technologists. SOA, layered over decision-support and selected transactional data stores, is gaining favor to increase agility. Another important technology is the operational data store, which can feed near real-time information via message-oriented middleware (MOM).
Executive Summary |
---|
Managing risk, always an essential business activity for companies in the insurance and financial services industries, is breaking out of its niche. With regulatory compliance touching multiple data sources and cross-functional business processes, risk management now requires an enterprise approach. More operations and lines of business face risk management scrutiny. And the kind of data, information and analysis generated by risk management has potential for strategic business objectives, such as improving customer profitability. Companies are blind to security, fraud and competitive threats if they can't use intelligent systems to bring the big picture of enterprise risk management (ERM) into focus. Relevant technology for risk management includes business intelligence, regulatory compliance tools, data warehousing and integration, message-oriented middleware, and business process management. Risk management will shake up existing distinctions between transactional and decision-support systems as organizations try to deal with problems close to the source and apply intelligence ahead of potentially damaging events and transactions. The first step toward ERM is to map architectural components to the types of risk that threaten your organization. Then, you can look at the timeliness and quality of data and information sources. An architectural approach will help you eliminate redundant efforts and match risk management with dynamic business needs. |
One large institution (preferring to remain unnamed) has layered a flexible reporting architecture over a high-performance data warehouse to give senior executives unparalleled access to risk data. Immediately after a large credit event (for example, something on the scale of Enron's bankruptcy), the chief risk officer could discover the bank's overall exposure to that customer within a few clicks on a Web page. At most banks, it would take weeks to get this information.BPM automation is essential given the quickening pace of business and the need for proactive risk management. In financial services, BPM automation plays a role in program trading, dynamic hedging engines, credit monitoring and limit trigger applications. A dynamic hedging engine, for example, needs up-to-date information about portfolio positions, which it will combine with market prices of traded instruments to perform risk calculations and execute trades — and then automatically rebalance the customer's portfolio. Organizations look to MOM to feed the hedging engines with timely data for CPU-intensive calculations.
Data repositories are broadly divided today into transactional and decision-support database systems. The former type support operational systems and are "deep but narrow" in data modeling terms. For example, a transactional database may have all the detailed information necessary for trade pricing, trading and settlement for a particular product or line of business. Data models are optimized for insert and update activity using the vocabulary of the business line.
Decision-support databases (usually data warehouses) tend to be "wide and shallow": that is, the information is standardized to make it comparable across business lines. These systems usually contain a lot of history. The risk management user base for decision-support systems has tended to be small, numbering in the tens or hundreds, with cyclical spikes in activity at the end of the month or quarter. However, growing interest in risk management is raising the intensity of performance requirements for these systems.
Data moves between transactional and decision-support systems typically via batch snapshots of business-line-specific aspects of the data. Often with the help of ETL tools, the snapshot extracts are transformed into standardized views. Data warehousing professionals know only too well the myriad data consistency and quality problems this process usually uncovers. However, transformation is the key because risk assessment, measurement and monitoring processes rely on timely, complete and high-quality information.
Drowning in Data, Dying for Information
Information is the set of explicit and unambiguous facts about the enterprise at any given point in time. Data, on the other hand, is merely the raw material of information. Data arises out of facts recorded throughout the enterprise, mostly by transaction systems. The process of transcribing facts into systems is corruptible: facts might be recorded inaccurately or spuriously — inadvertently or with malicious intent. Risk management architecture must prevent, or at least be able to unwind, data corruption to deliver quality information.Banks struggling with Basel II compliance have discovered gaps in how they collect required transaction data, but the most pressing problem is translating that data into useful decision-support information. Inconsistent data semantics and poor data quality are the stumbling blocks.
Thus, organizations are formulating data governance frameworks that encompass a mix of technology, processes and policies covering all aspects of data management, including metadata consistency and data quality improvement. BI tools, ETL engines and metadata repositories are among the relevant technology solutions pointed at these problems. Data governance frameworks must define the links between transactional and decision-support systems; they must show IT how to detect quality problems and address them at the source rather than through data cleansing procedures.
Illuminate the Unknown
Spurred especially by regulatory compliance, risk management has come a long way. Financial services organizations, for example, have become reasonably adept at managing market and credit risk. Operational risk is the new frontier. As organizations learn the precise drivers of operational risk, the ERM architecture and its attendant analytics will extend into more aspects of how a company operates.
Risk integration — the attribution of losses by risk type and the study of the interplay between them — is another growing area of interest. If a market portfolio suffers a drop in a company's bond prices due to a reduction in the issuer credit rating, for example, should the company look at the event as a market loss, credit loss or both? Answering such questions requires sophisticated analytic engines and increased integration between risk management systems.
Finally, risk management is increasingly an important source of knowledge for competitive advantage, a subject covered in depth in the next article in this cover package. Recent interest in capital measures (due partly to Basel II and similar initiatives) has fueled interest in using industry risk measures to determine risk-based pricing. Information on capital, best calculated and allocated in enterprise data repositories, must be fed to transactional systems where products are priced. This "reverse flow" of information from decision-support to transactional systems has huge implications for how both kinds of systems will be developed and deployed. Decision-support databases must step up to OLTP-level performance, availability and security requirements.To succeed in risk management and discover new business opportunities with risk information, we must shine a brighter light on the ERM business function and its supporting architecture. As interest in risk analysis grows, distinctions between data repositories will blur. Organizations must formulate an architectural view so they can handle not only unknown risks to the business but also unknown requirements for information transformation.
R. Dilip Krishna, a principal with ProRisk Group, has more than 15 years experience in technology and business consulting. He has worked on risk management and Basel II implementations at several large North american banks and was chief architect of the Basel II program at CIBC. His current focus is on enterprise risk warehouses. Write to him at [email protected].
You May Also Like