Elevators Vs. Networks: Applying Predictive Analytics
Could predictive analytics help foresee network failures?
August 8, 2017
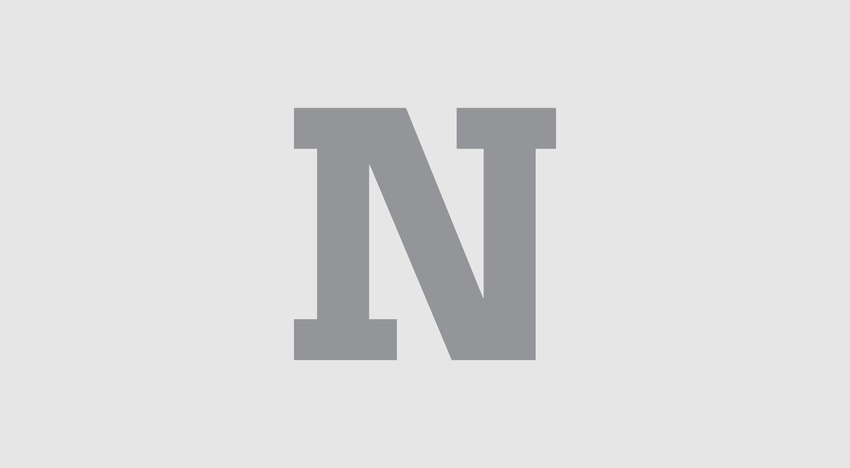
I was recently watching a television ad that made me think of how predictive analytics can be applied to networks. The ad showed a repairman entering an office building and saying, "I'm here to fix the elevator." The security guard responds, "We have no problem." Then the repairman replies that the problem will occur in three days, and he was able to know this through access to IBM Watson performing predictive analysis.
In regard to networks, could predictive analytics allow us to predict network problems such as failures, degradation, and performance issues? It seems a reasonable question to ask, however, it requires some knowledge of analytics technologies to understand the success of predictive analytics for networks.
The elevator vs. the network
The elevator is part of the building. It has its own architecture and infrastructure. Most of what happens to the elevator as it goes up and down is repeated every day. The elevator's environment does not change much unless there is a fire, earthquake, or some other factor which affects the elevator's operation. There is a long history of the elevator operations within the building. There is even a greater history of that same type of elevator operating in other buildings available to the elevator manufacturer.
The network is a different story. The network may change based on redesign, traffic behavior, routing changes, application changes, and software modifications. The network is not as static in its operation when compared to the elevator.
Service providers are trying to implement Network Function Virtualization (NFV) and Software-Defined Networks (SDN), which change provisioning, billing, and operations. One of the biggest changes will be the ability for an enterprise to demand network and capacity changes to be delivered in minutes rather than weeks. This makes it harder to predict network operation because the data collected is about a dynamic (not static) network. There may be very little historical data available after the customer makes changes to the network's operations. Less data means weaker predictions.
Predictive analytics foundation
Predictive analytics incorporates machine learning (ML) and artificial intelligence (AI) processes. AI can be implemented in any device that measures its environment (network), and takes actions that maximize its chance of success for a goal such as predicting network operation. AI is applied when a machine mimics human functions such as learning and problem solving. ML gives computers the ability to learn from data without being explicitly programmed in advance.
Predictive analytics has improved, benefiting from advances in AI and related fields. IBM's Watson is an example of this implementation process. The limitation of AI is that the accuracy of the predictions will depend on the quality the data collected.
Read the rest of this article on No Jitter.
About the Author(s)
How to Amplify DevOps with DevSecOps
May 22, 2024Generative AI: Use Cases and Risks in 2024
May 29, 2024Smart Service Management
June 4, 2024