Big Data's Evolving Role in E-discovery: What Is Predictive Coding?
Predictive coding is helping to speed up the often laborious and intense e-discovery process. Learn more about the technology, and why organizations are still taking a measured approach.
August 17, 2012
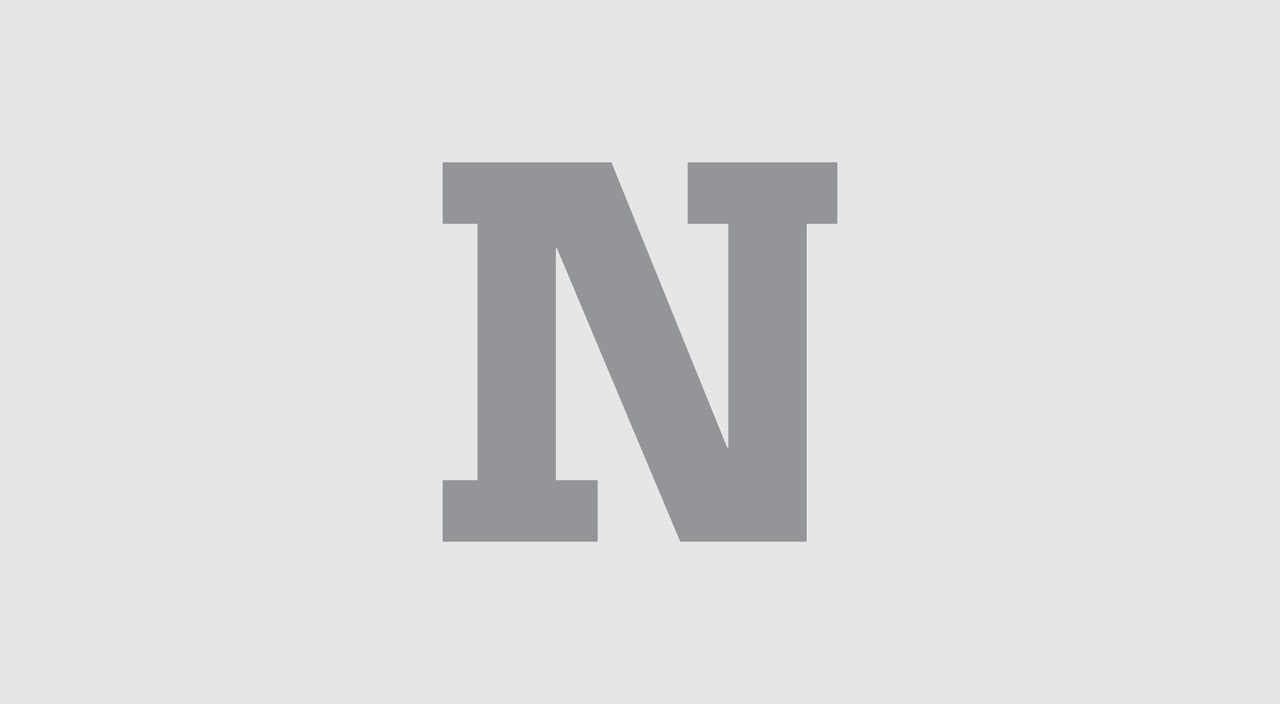
Trying to understand big data recalls the story of the blind men touching an elephant. Although we don't have a clear picture of the totality of the big data elephant, we can still learn something from each part that we touch. Understanding the role of big data in e-discovery is one such example.
(Note that the following discussion focuses on the use of predictive coding, which is an analytical technology for processing e-discovery "big data." Since it's a data-driven intelligence software application, "predictive coding" is also the name for a class of products that all try to accomplish the same goal. For purposes of this discussion, predictive coding will be used in a general sense.)
The purpose of civil litigation is to determine who wins a lawsuit and who loses money. However, the monetary impact can be found in more than the potential monetary awards--there's also the cost of the litigation process itself. That can be quite expensive, especially when hundreds of thousands or even millions of documents (sometimes even tens of millions of documents) are involved in large cases. So keeping e-discovery costs down is a key goal of an enterprise's internal legal team.
As part of the e-discovery process, the team must determine which documents are responsive (relevant to the litigation). All documents must be examined and winnowed down to (hopefully) this much smaller subset. The process was previously done manually, which has been a reasonable approach, although human beings are prone to error (especially when scanning a lot of documents) and reasonable people can disagree. It was costly, however--after all, this isn't a minimum-wage situation, and even outsourcing is expensive.
A second approach is to use keyword search. While this sounds useful and can be of some help, FTI Technology, a leading e-discovery vendor, reports that generally only a fraction of responsive documents can be found via keyword methods.
Predictive Coding: Predictive Analytics for E-discovery
An advanced analytical approach called predictive coding can now be used to successfully winnow a set of documents. A small subset of all the documents--enough to provide a statistically reliable sample size--is examined manually, and the documents are classified as responsive or nonresponsive. Different predictive coding schemes exist, but the algorithms and heuristics apply their artificial intelligence, machine learning, data mining or whatever you want to call it to classify documents that are considered responsive in a civil litigation case.
That sounds great, but when potentially very large sums of money are involved and traditionally accepted, andhuman-based processes are being dramatically altered, questions legitimately arise, such as the following:
Can lawyers effectively defend the use of predictive coding?
Will the courts accept the use of the technology?
For what type of legal matters is the technology well suited, and for what types is it not?
Are the economic cost savings real enough to justify the use of the technology?
How is the adoption process proceeding?
Next: Survey Finds More Inclined to Use Predictive CodingTo get a better understanding of those and other related questions, FTI Technology commissioned a survey of 24 in-house and law firm counsel. Some of the results from the report, "Advice from Counsel: Can Predictive Coding Deliver on Its Promise?," include the following:
• Emerging case law that supports the use of predictive coding has led more than half of the respondents to say that they're more likely to use it. As an analyst, I have been pleased and surprised that courts and attorneys who tend to have a different logical thinking process than, say, business intelligence analysts have generally supported the use of e-discovery technologies, of which predictive coding is just one example.
• Predictive coding is a process, not just a technology, and people have a critical and vital role that can result in the successful use of the technology. People have to determine the reference set (that is, the test set of data used as a training vehicle for predictive coding); refine the software; and conduct the necessary quality control to ensure defensibility.
• Although predictive coding can deliver huge savings, the verdict is still out on whether the savings exceed the additional costs, such as software. Quantifying costs and savings turns out to be quite difficult. More work needs to be done to define calculations and how they would apply to types and sizes of cases for which predictive coding would be appropriate.
• Predictive coding would tend to be most appropriate in situations involving 100,000 or more documents or in particular types of matters, such as class-action suits or cases mandating large-scale reviews in short time frames, such as those conducted by the Federal Trade Commission or the Department of Justice. Predictive coding isn't as appropriate in small-volume cases, where the documents aren't text-based, such as photographs, images or audio files, or where trying to find the proverbial "needle in the haystack" is the target of the investigation.
• Organizations are taking a measured approach to the adoption of predictive coding--many don't want to be early adopters, but that hasn't prevented a little more than half from using or trying it. A deliberate and sensible approach doesn't mean that predictive coding won't be successful, just that we're still in the early-adopter phase, rather than the early-majority step of the process.
The bottom line is that predictive coding seems like a valid process technically, but its complexity suggests a deliberate, pragmatic adoption process.
The primary market research survey conducted by FTI Technology gets into the heart of the issues that affect the adoption of predictive coding. Although this is only one illustration of big data and its associated analytical technologies, the lesson that can be learned is this: Carefully think through the drivers and inhibitors that affect the adoption of a technology, so that you can take the appropriate actions.
FTI Technology is not a client of David Hill and the Mesabi Group.
About the Author
You May Also Like