EMC Sees Big Opportunity In Big Data
According to an EMC-sponsored IDC report, the amount of data amassed by consumers and businesses is expected to increase by 44 times in this decade. A lot of that information will be what many, including EMC, call big data. Obviously, big data requires storage and other products and services that the company provides, so it should come as no surprise that, in its recent blizzard of announcements, EMC targeted big data as one of its key markets. Let's try to understand big data and what it means,
February 14, 2011
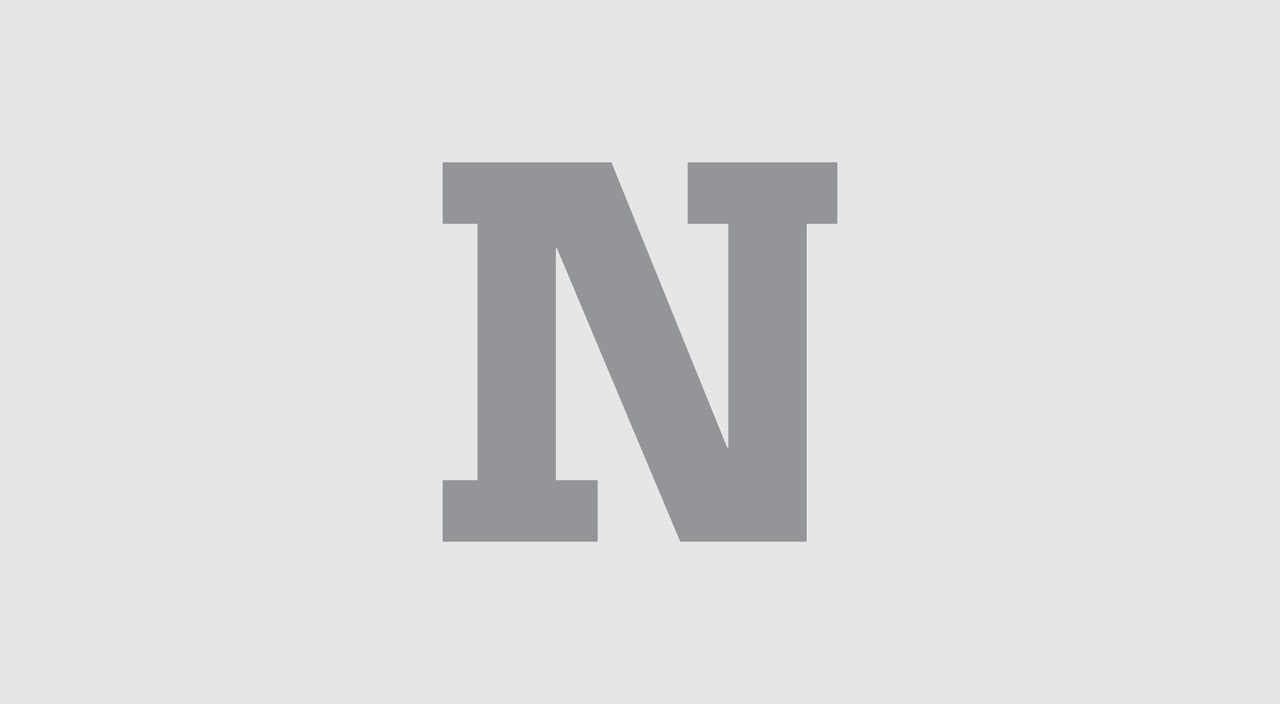
According to an EMC-sponsored IDC report, the amount of data amassed by consumers and businesses is expected to increase by 44 times in this decade. A lot of that information will be what many, including EMC, call big data. Obviously, big data requires storage and other products and services that the company provides, so it should come as no surprise that, in its recent blizzard of announcements, EMC targeted big data as one of its key markets. Let's try to understand big data and what it means, and then briefly illustrate how EMC is addressing the big data market through its recent acquisitions of Isilon and Greenplum.
EMC's working definition of big data is "data sets, or information, whose scale, distribution, location in separate silos or timeliness require customers to employ new architectures to capture, store, integrate (into one data set), manage and analyze to realize business value." Now, that is quite a mouthful and requires some time to digest and, of course, it fits around what EMC can or wants to do. However, the definition covers the essence of the subject and makes some valid points. But let's look at some examples to gain a better perspective on the breadth of where big data resides in the real world:
Medical information--including medical images, such as MRIs, as well as electronic health records (EHRs);
Increased use of broadband on the Web--including the 2 billion photos each month that Facebook users currently upload, as well as the innumerable videos uploaded to YouTube and other multimedia sites;
Video surveillance--this is a booming business with a need for enormous volumes of storage, as well as the advanced analytics to make sense of it;
Increased global use of mobile devices--the torrent of texting is not likely to cease;
Smart devices--sensor-based collection of information has a tremendous future enabling smart electric grids, smart buildings and many other public and industry infrastructures;
Non-traditional IT devices--including the use of RFID readers and GPS navigation systems;
Non-traditional use of traditional IT information, including the transformation of OLTP into, say, a data warehouse for applying analytics, e-discovery and Web-generated information tools; and
Industry-specific requirements, including high-performance computing solutions in genomic research, oil and gas exploration, entertainment media, etc.
Now, a critic might say that there is nothing new here. For example, medical images and broadband Web access have been around for a long time. The reply is that big data-related changes are probably mostly a matter of degree but also, to some extent, a matter of kind. The matter of degree comes about because of the increased intensity of usage and higher scale--sheer volume of petabytes of storage--than we have ever had. The matter of kind relates to the transformation of data from analog to digital and the need to get business value in new ways. But the key point to remember is that big data is a huge market that translates into "big money." From an IT business perspective, that is why big data matters.
There have been, roughly speaking, three major waves in the kind of structure that information has had from an IT perspective. Note that these new waves do not replace the old waves that continue to grow, and all three types of data structure have always been present, but one type of structure tends to dominate the others:
Structured information. This is the information that finds a home in relational databases and has dominated the use of IT for many years. It is still the home of mission-critical OLTP systems businesses depend upon; among other things you can sort as well as query on structured database information.
Semi-structured information. This was the second major wave in IT and includes e-mails, word processing documents and a lot of information stored and presented on the Web. Semi-structured information is content-based and can be searched, which is the raison d'etre of Google;
Unstructured information. This can be thought of as primarily bit-mapped data in its native form. The data has to be put in a form that can be sensed (such as seen or heard in audio, video and multimedia files). A lot of big data is unstructured, and its sheer size and complexity require advanced analytics to create or impose structure that makes perceiving and interacting with it easier for humans.
Unfortunately, this classification scheme is not perfect. First, there are numerous hybrid and composite forms such as a photo embedded in a word processing document. Secondly, while "records" is a term that applies to databases, and much of the semi-structured information is stored in files, other information resides in streams such as captured by a video camera. And then there is the entirely separate concept of objects.
The bottom line, though, is that traditional IT infrastructures--including servers, storage and networks--were built around structured information and bent to adapt to semi-structured information. However, they are really not designed for the multifaceted structure requirements, scale and analytical demand required by big data.
That is why EMC underlined new architectures in its definition of big data, and that is also why it acquired Isilon and Greenplum. Much has been written about these acquisitions, so I will focus briefly on how the companies illustrate the need for different architectures for big data.File-based storage, on which a lot of big data applications is based, is growing at a much faster rate than block-based data. IDC predicts that 80 percent of all storage capacity sold will be for file-based data. Network attached storage (NAS) is often used with file-based data, but scale-up NAS had its limitations on a number of dimensions, including scalability and performance. A scale-out NAS storage architecture overcomes these limitations.
For example, Isilon's scale-out NAS architecture that uses its OneFS operating system can scale up to 10 plus petabytes in a single file system and support up to 50GBytes per second of throughput. However, big data applications may emphasize one dimension or the other of the data involved. Consequently, Isilon sells the S product series purpose-built for high-transactional and IOPS-intensive applications such as genome research, while the company's X-Series solutions are targeted at capacity-intensive applications, such as those that need to handle high-concurrent and sequential throughput applications, such as medical images.
Greenplum focuses on the analytical challenges posed by big data. Its suite of products supports big data sets that are analysis-intensive, ultimately helping end users glean salient insights from their data. This typically requires complex analysis, such as ad hoc, interactive analysis, and not simply the production of structured reports. The speed of analysis is important especially if it needs to be performed frequently and when insights facilitate decision-making.
However, traditional relational database management systems are not optimized for big data analytics. Remember that they were designed to meet the small random reads and writes required by OLTP rather than the sequential reads an SQL query may demand. To meet those different needs, Greenplum developed a massively parallel processing (MPP) system, where performance and scalability are key elements. Again, Greenplum illustrates a new architecture that is needed to meet big data application requirements.
Big data applications come in many flavors, but one constant is that they typically consume vast amounts of storage. Scientific and engineering uses of big data, such as in high performance computing (HPC) scenarios, have been around a long time, but now big data is spreading into mainstream information technology, including entertainment media, health care and the Web.New mainstream big data IT applications tend to capture or create data through mechanized electronic and electromechanical devices, such as medical equipment, cameras, RFID readers and sensors, rather than through the use of human touch or voice. In general, this data tends to be much less structured than human-created information, which leads to structured or semi-structured information.
Whatever the level of structure, the ability to make sense of massive amounts of data to generate insights that can serve as the basis for decision-making tends to require new and higher levels of analytical tools. Moreover, the scaling requirements on both performance that asks "How fast the data can be processed?" and capacity that asks "How much data can be efficiently supported" tend to be different than those supported by traditional information technologies. Altogether these different requirements tend to require new architectures.
EMC, as well as many other companies, see big data applications as a big money market. So it should come as no surprise that EMC has invested in both Isilon and Greenplum in order to help it address the opportunity that big data presents. Although no company that makes numerous acquisitions can have a perfect track record, EMC has time and again demonstrated that it has a "green thumb" for acquisitions. One of the reasons is that it values the people who join as part of the acquisition. It not only listens and encourages them, but also adds its own expertise, financial resources and established distribution channels to positively augment what the acquired companies already had.
And both Isilon and Greenplum were well-respected independent companies whose technological prowess was demonstrated by their large customer bases. Big data customers should expect that EMC will continue to add features and functions to the base technologies that it acquired, and the customers of those companies will have to carefully examine what EMC brings to the table--and that is a lot.
EMC is currently a client of David Hill and the Mesabi Group.
About the Author
You May Also Like