Big Data Means Big Storage Choices
It's tough to keep up with what big data you'd like to store, especially when much of the data is unstructured text from outside--perhaps from blogs, wikis, surveys, social networks, and manufacturing systems.
July 26, 2012
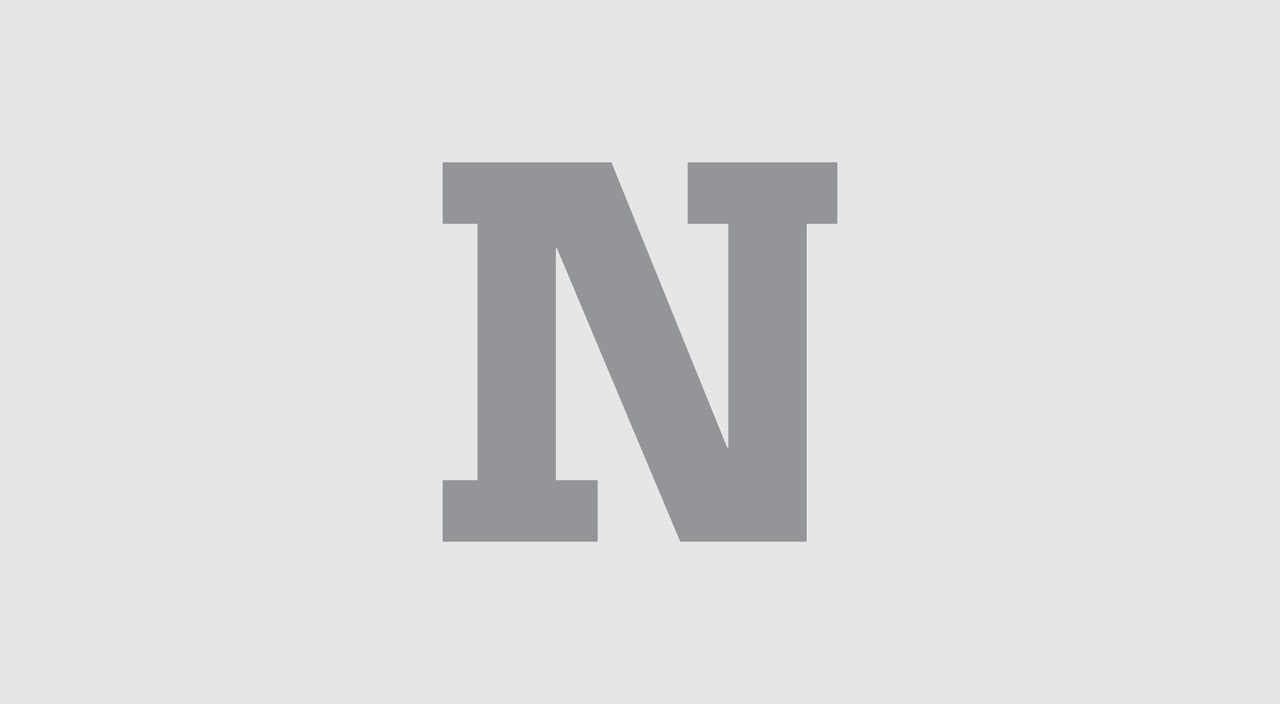
Big Data Talent War: 10 Analytics Job Trends
Big Data Talent War: 10 Analytics Job Trends (click image for larger view and for slideshow)
Big data can improve the operational efficiency of companies using it by as much as 26%, according to a report released this month from Capgemini North America. That's a huge leap that will grow even larger--to 41%--within three years, if opinions of the 600 C-level executives and senior IT people Capgemini surveyed for the report are to be believed.
Two thirds of respondents said big data will be an important factor in business decisions, and will accelerate decision-making processes that have been slowed by excessive, inefficiently managed data. Eighty-four percent of respondents said the goal is to analyze big data in real time and act on it immediately to keep on top of changes in the market.
So why hasn't big data taken over the market for customer-behavior analysis and marketing? It has, at least to the extent most companies can manage it, according to analysts. Big data, like cloud computing, is a technology reference only by fiat; there is no "big data" SKU an IT department can order to get into big data management. There's not even a common definition. Any data a CIO can manage that directly affects top-line revenue is data that is valuable, no matter what its size, said Forrester analyst Vanessa Alvarez.
[ Read Feds Face 'Big Data' Storage Challenge. ]
"Big data means big value," she said at the May Interop show in Las Vegas. The problem with big data isn't defining what it is; the problem is in keeping up with what you'd like to store, especially when most of the data that becomes "big" is unstructured text from outside the company--from blogs, wikis, surveys, social networks, and other sites as well as operational data coming in from intelligent monitors built into manufacturing and transportation systems, said Alvarez.
However valuable the insight from Big Data, every project comes with a major downside: the cost of "big storage".
Traditional databases don't write or process data fast enough to handle giant pools of data, which is why the open-source database Hadoop has become so popular, according to John Bantleman, CEO of big data database developer RainStor, in an article for Forbes. An average Hadoop cluster requires between 125 and 250 nodes and costs about a million dollars, Bantleman wrote. Data warehouses cost in the tens or hundreds of millions, so Hadoop delivers the goods at a huge discount. When you're talking about data sets such as the 200 petabytes Yahoo spreads across 50,000 network nodes, you get into real money.
In March, IDC released the first projection of the worldwide market for big data. It predicted the market would grow 40% per year--about seven times as fast as the rest of the IT industry. Most of that cost, or at least the biggest part, will come from infrastructure-investment-caliber storage projects that will drive spending in the storage market to growth rates above 61% through 2015, according to IDC analyst Benjamin Woo.
The data sets themselves are growing as well. Though most big data sets are not overly large yet, they are growing in size by an average of 60% per year or more, according to IDC. The result, according to a February Aberdeen Group report, is that many companies will have to double the volume of their data storage every 2.5 years just to keep up.
Data compression and deduplication can reduce the amount of storage required by almost a third and data tiering can reduce per-unit costs by putting data in low demand on low-cost media such as DVDs or tape. The most effective way large companies deal with out-of-control data growth, however, is with scale-out NAS deployments whose costs rise much more slowly than those of more sophisticated storage area networks, whose costs rise linearly with the volume of data stored, Aberdeen concluded.Many companies, especially mid-size ones, have avoided some big data projects because the high-end, high-performance storage they specify as standard for those projects costs half a million dollars to store 20 to 40 terabytes, according to an interview consultancy Sandhill Partners did with Fred Gallagher, general manager of big data cloud developer Actian.
Actian's main product, Vectorwise, scales more efficiently as users add more processing cores than it does simply adding more servers. That approach--making relatively inexpensive storage hardware perform up to the level of its more-expensive relatives--is the more effective way to scale storage networks to keep up with data that gets bigger and bigger ad infinitum, Gallagher said.
Scale-out NAS boxes do much the same thing: they make big data projects with budget-busting levels of growth slightly more palatable, or at least more affordable.
Dell launched a big data storage package July 23--a rack of products based on Apache Hadoop, that starts with two TB of storage and ranges up into petabytes. The product includes Dell's Cloudera data-management software, Apache Hadoop, Force 10 networking, and Dell PowerEdge servers. It also includes data compression technology capable of shrinking data at ratios of 40:1. This frees up disk space, reduces the number of units needed for a big data project, and saves customers money—but they still must spend vast amounts on storage for data that, in most cases, has yet to prove its worth.
It will, predicts Alvarez and other analysts.
Massive amounts of data and the ability to analyze it quickly enough that the results are still useful are so important as decision-making tools that data forms the fourth paradigm of computer science – a whole new way of considering, analyzing, and making use of data, according to computer scientist Jim Gray, who just published a book of essays called The Fourth Paradigm: Data-Intensive Scientific Discovery.
The first three paradigms, according to the British computer scientist Amnon Eden, differed radically in the assumptions with which they approached computers. The first treated computers as a branch of mathematics in which applications were formulae designed to produce a practical result. The second treated computer science as an engineering discipline and programs as data. The third, the scientific paradigm, treats applications as processes on a par with those of the human mind, an approach that assumes programs will eventually develop their own intelligence.
The idea is a little abstract for IT, but each paradigm brought with it a new way of analyzing problems: first according to observation, then by theory, and finally by simulation. Big data goes beyond all of those by promising to deliver insights so integrally concealed in massive amounts of data that direct observation and analysis by humans can never coax it out. Finding those answers requires enough data to make indirect correlations clear, however. Having enough data to mine for indirect correlations requires having enough storage hardware to house all that data and access it quickly.
Having that much storage hardware--there's no other way to say it, according to Alvarez, and Woo--means spending a lot more money on storage, no matter how efficiently it can be made to run or how cheaply it can be bought.
Big data places heavy demands on storage infrastructure. In the new, all-digital Big Storage issue of InformationWeek Government, find out how federal agencies must adapt their architectures and policies to optimize it all. Also, we explain why tape storage continues to survive and thrive.
About the Author
You May Also Like