Big Data For IT Operations: Data Lakes Or Data Warehouse?
IT operations itself can benefit from the promise of big data analytics, but choosing the right data storage ecosystem is essential.
May 7, 2015
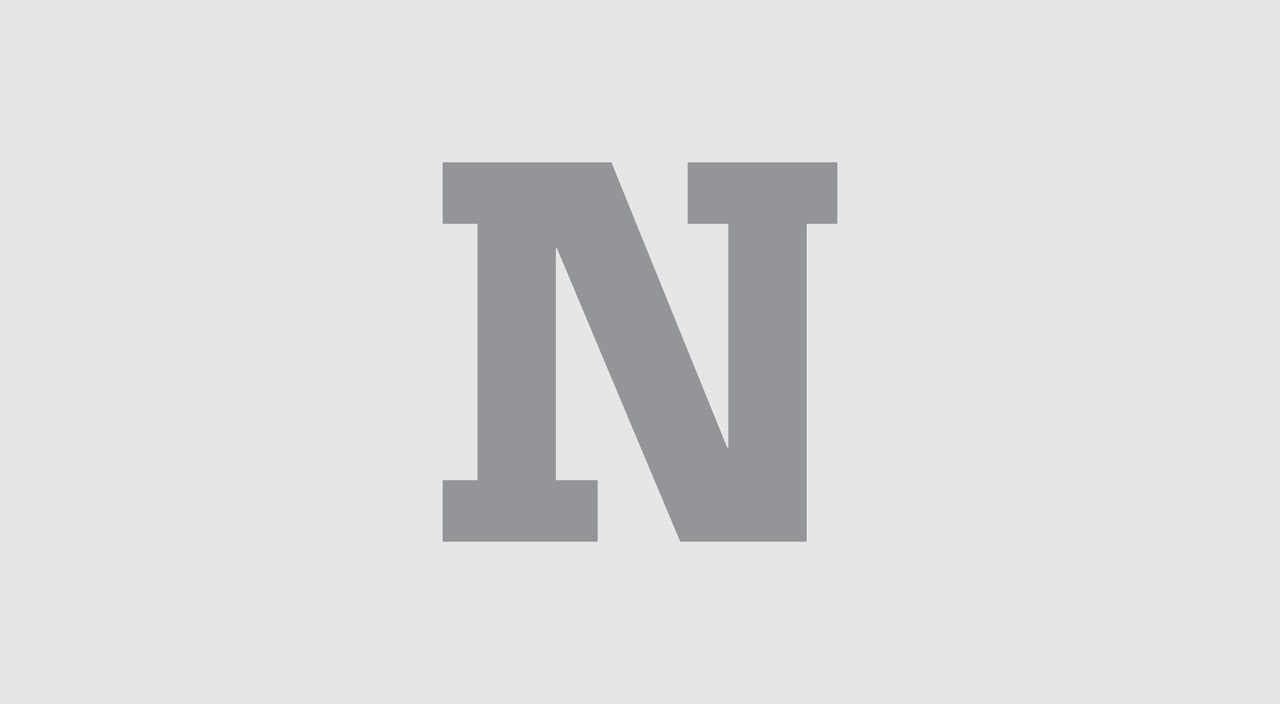
We are solidly in the midst of the era of big data, along with the big hype that goes with it. The next generation of analytics platforms has been emerging for the last few years, but as we enter the implementation phase, the real work begins.
There are a lot of vendors and new tools in the market now, but most experts are predicting consolidation. Likewise, companies that want to use analytics programs to stay competitive are pushing to get beyond the beta phase and start reaping rewards from established, well-planned initiatives.
Among the most practical use cases for big data analytics is the innovative mining of IT operational data. IT departments have long been tasked with collecting data in service of automating and optimizing all kinds of business processes. I see this turning inward as more IT teams are collecting petabytes of raw machine, sensor, and log data in hopes of visualizing and optimizing their own operations.
Doing anything meaningful with such massive amounts of data is challenging. An ecosystem of IT operations management solutions is bubbling up around the use of open source, Hadoop-based data lakes. As the technology matures, enterprises are moving from storage and batch analytics to streaming, real-time data processing built on flexible, modular platforms. Because early adopters have won visibly significant competitive advantage through their data initiatives, analytics are going mainstream, spurring a new wave of innovative solutions.
While the Hadoop ecosystem is not suited to every type of data project, it points the way to the intelligent liberation of data. To make big data self-service, it has to be accessible to business end-users, not just data scientists and datacenter gurus. To unlock the true potential of big data, the sharing of large data sets has to become more lightweight and transparent. Solutions that address high cost barriers to entry, vendor lock-in, and ultra-rigid data should have a democratizing effect.
As the use of data lakes increases, so do the concomitant challenges. Many are asking why and when it makes sense to deploy a Hadoop-based system instead of an enterprise data warehouse (EDW) model. Gartner has cautioned that data lakes can easily become data swamps, full of dirty data and stagnant from lack of use.
As always, security, privacy, and compliance concerns are front and center; making a Hadoop environment ready for sensitive information requires custom hardening and configuration. Hadoop-based deployments still require hardware and software installation and management; and new skill sets are needed to integrate modules and applications like Hadoop Common, HDFS, YARN, MapReduce, NoSQL, and analytic discovery.
Data lakes are an easier and faster way to park and process massive amounts of unstructured data from multiple sources; the most salient feature of Hadoop is that it doesn’t require schema-on-write. This is a timely solution for companies that know they have a lot of valuable data but aren’t quite sure what to do with it yet. Data scientists will also benefit greatly from running experiments in such an open and evolving framework.
But depending on the data type, use case, or desired outcome, the lack of structure can be a major drawback. The information being added to a data lake carries no metadata, and without a modicum of curating and governance, it is hard to determine the quality and provenance of the data.
Data warehouses, on the other hand, sanitize and organize data upon entry, enabling consistent and predictable analysis across pre-categorized structures. The ability to replicate standard queries and reports over time across uniform datasets is essential to many enterprises. In other words, data warehouses provide value that will not be replaced by data lakes, no matter how flexible they are.
With either approach, and regardless of which platform or tools you ultimately deploy, getting the basics right is essential. Storing and accessing data elegantly doesn’t necessarily solve business problems or boost the bottom line.
Measuring and analyzing the right things, asking the right questions, and involving the right stakeholders are always keys to success. How do we know if we are measuring the right things? This is where IT and business leaders must cooperate and keep the focus on business needs. Once a meaty business problem has been identified and assessed, it is easier to pick which tools are better suited to building a solution. Sometimes it will involve analyzing data in rigid silos; sometimes it will be drawing samples from a fluid pool of data.
As next-generation analytics continue to evolve, we will no doubt invent new approaches that blend these models to achieve even deeper levels of knowledge, to the benefit of the data center and beyond.
About the Author
You May Also Like