The Real Source of Cloud Overspend? The Shift from CapEx to OpEx
Even with the most dedicated attempts to curb cost, there are inherent features of the cloud landscape that make managing cost difficult.
February 6, 2020
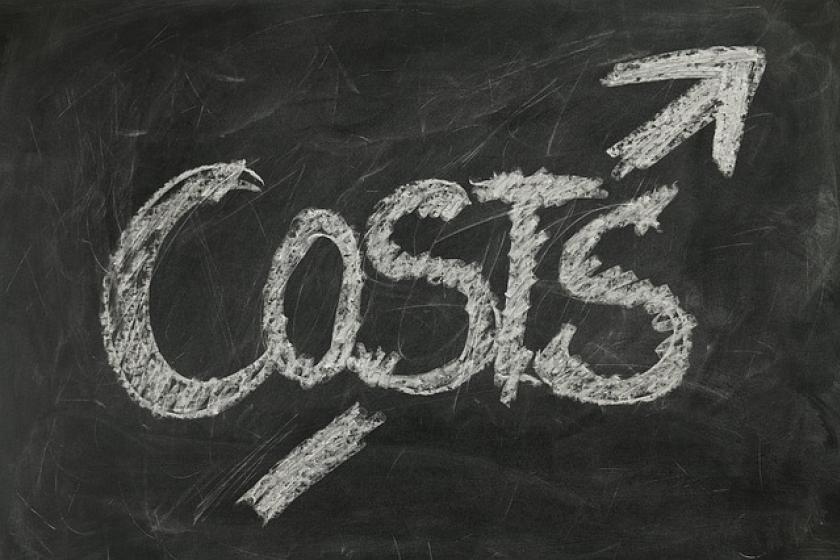
The rise of the cloud has changed the face of Big Data. Whether through lift-and-shift or re-architecting, almost every modern enterprise is now managing a hybrid, and usually a multi-cloud Big Data environment.
The problem? The shift to a hybrid environment has created a cost crisis. When enterprise IT organizations receive their first few cloud bills, many are shocked. Bain & Company asked more than 350 IT decision-makers what aspects of their cloud deployment had been the most disappointing. The top complaint was that the cost of ownership had either remained the same or often increased.
Gartner estimates that “through 2020, 80 percent of organizations will overshoot their cloud IaaS budgets due to a lack of cost optimization approaches.” 80 percent!
The CapEx to Opex challenge
What happens when large-scale cloud migration begins? In an on-prem data center, there is an inherent and internal limit to compute capacity. An on-prem data center will never double its capacity overnight. Any utilization gains are hard-won, and IT teams can struggle to free up resources to meet business demands.
The cloud is seen as the obvious solution to this problem. With AWS, Azure, or Google Cloud, you face none of the baked-in limitations of an on-prem data center. The technical and internal bottlenecks of the legacy architecture vanish.
However, the legacy, on-prem data center operated within a CapEx model. Though the tech was constrained, so was the budget. But as the infrastructure migrates to the cloud, a CapEx model is exchanged for an OpEx model. And here’s where the trouble starts.
In the CapEx framework, the balance sheet was very clear, and projections were simpler. Traditionally, the CFO would oversee strict cost control mechanisms. Though this translated to constrictions on compute capacity, the trade-off was watertight budgeting.
But in the cloud-based OpEx paradigm, the control flows of how money is being spent suddenly become much looser and harder to define as there is no hard-coded capacity ceiling. For every internal team, an all-you-can-eat approach to resources sounds like the promised land.
An OpEx spending model plus the infinite resources of the cloud equals a recipe for overspending. Suddenly, an engineer can spin up a hundred-node cluster in AWS on a Friday, forget about it, go home, and discover a month later that it racked up thousands in cloud costs.
How to gain control in an OpEx model
Controlling spend in an OpEx model requires one thing: visibility.
Even with the best cloud migration strategy, and even the most dedicated attempts to curb cost, there are inherent features of the cloud landscape that make managing resources – and therefore cost – much more difficult.
In a large system, hundreds of thousands of instances will be supporting thousands of workloads, all of which are running big data computations for a range of internal customer teams. The range of ways to provision resources and compose the instance is much larger in the cloud than in a legacy architecture. With so many live instances, the implications for cost can be very hard to track.
The answer? Workloads need to be rightsized. And the key to rightsizing lies in visibility. You need to determine usage patterns, understand average peak computing demand, map storage patterns, determine the number of core processors required, and treat nonproduction and virtualized workloads with care. To stay rightsized post-migration, you need full insight into the actually required CPU, memory, and storage.
Get the software you need
This sort of visibility is what can give people an understanding of what cloud costs they are generating. However, the data and insights that IT operations teams need are almost impossible to acquire without the right tool. Even if they had the expertise, most organizations don’t have the human resources or hours to dedicate to reducing cloud spend in a granular way. This would require expertise and time. Even someone with the skills would be playing a whack-a-mole of workload management.
IT leaders need visibility to determine usage patterns, understand average peak computing demand, map storage patterns, and determine the number of core processors required. They need software that can take a targeted approach to rightsizing by identifying wasted, excess capacity in big data cluster resources. By monitoring cloud and on-premises infrastructure in real-time, and by leveraging machine learning with active resource management, they can automatically re-capture wasted capacity from existing resources and add tasks to those servers.
Related articles from the Network Computing archives:
About the Author
You May Also Like