The Challenge Of Making Business Intelligence And Analytics Work
Introducing "smart" systems and business analytics into an organization is an IT challenge. Historically, IT has supported online transaction processing for internal operations and customer-facing systems, and then used batch processing to wrap up all of the activities at the end of a processing period. But increasingly, businesses are beginning to incorporate smart systems that go beyond isolated enclaves of automation built into computers and networks and tap directly into the critical thinkin
October 26, 2011
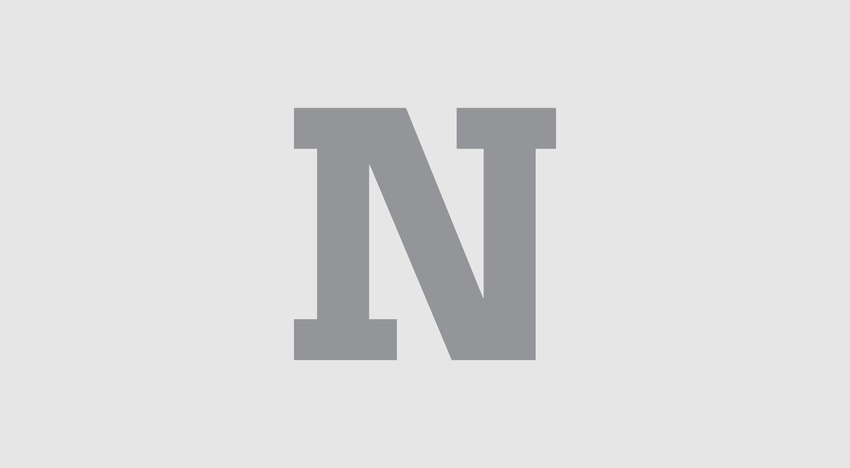
Introducing "smart" systems and business analytics into an organization is an IT challenge. Historically, IT has supported online transaction processing for internal operations and customer-facing systems, and then used batch processing to wrap up all of the activities at the end of a processing period. But, increasingly, businesses are beginning to incorporate smart systems that go beyond isolated enclaves of automation built into computers and networks and tap directly into the critical thinking behind mission-critical business processes.
To cite some examples:
Retailers like Amazon monitor traffic on their websites to determine the Web pages most frequently visited and also to log demographic information from sales so they can tailor product pitches to individual consumers.
Professional football teams like the Seattle Seahawks consolidate ticket sales transactions with customer demographics in a single database so that intelligence can be gathered and analyzed for the delivery of custom promotions to highly targeted segments of their customer base.
Primerica builds in business intelligence and automation to "bullet-proof" the insurance application process that its 95,000 in-field independent sales agents step through with clients to ensure that the process goes smoothly and correctly the first time.
SUSE Linux develops a tool that can create a virtual instance of a Linux image for virtualization that automatically checks that image for compatibility with the current Linux operating system and with IT’s compliance and other business rules--and with the click of a mouse, sends that image to any target platform---from an x86 computer all the way up to a mainframe.
A major European metro subway records and stores video camera images in a database, and uses an analytics software package to monitor tracks and tunnels for any abnormal visual pattern—such as a package that seems to be sitting too long in a particular area that may need to be investigated.
A package delivery company uses analytics software in conjunction with GPS and the digital tracking of trucks to evaluate when trucks are taking too long to run their routes and why.
A major supply chain sourcer analyzes available shipment options for least cost and fastest delivery on a 24/7 basis, and makes at-the-moment, real-time logistics decisions.
The list goes on and crosses every industry. For IT, it means making infrastructure and technology performance decisions that can accommodate the business’s demands for the same real-time performance from its analytics and decision support systems as it has demanded in the past from its transaction processing.
How do you get there?
Servers--If you are going to infuse the business with real-time analytics, you can’t get there by reprovisioning servers that were purchased and deployed for processing large volumes of transactions because analytics processing is a different function. One reason is that transaction processing servers read data in rows instead of in columns. As Sybase director of analytics Don Lahl pointed out, this is like "entering a UPS truck in the Indy 500." Analytics servers do read data by rows, but most of the time they drill down for information by looking at columns of data that contain data attributes such as "sales" to point you to sales data. Reading by column instead of by row is faster and more expedientfor retrieving query information because you’re bypassing data topics that don’t matter to your query.
Databases—IT often finds itself engaged in large-scale data cleanup and also in database consolidation projects before it can even begin to pursue the concept of an effective analytics database. Once the analytics database is established, there are issues of data concurrency, consistency, staleness, and so on that still have to be resolved by IT to assure that the results of both transaction processing and analytics servers and databases are synchronized to the real-time world that the business wants to see and respond to. Today, this synchronization process is manual, but more analytics vendors are looking at system automation and server integration solutions that are likely to help in this area.
Throughput, priority setting and resource allocation—Most organizations have a history of running analytics (formerly known as decision support) at a low priority during the day, and then executing many of the requested queries and reports during the nightly batch processing window. However, real-time analytics for at-the-moment decision-making and operational automation can’t work like that. This leaves decisions for IT as to how it is going to modify its technology infrastructure, priorities and workload bandwidth in order to keep both analytics and transaction processing running at high priorities.
Failover—Historically, decision support systems were deemed to be non-mission-critical in disaster recovery and failover scenarios, For the immediate future, this will remain so for most companies. However, as more automation and instantaneous business response is demanded, this could change.
Clearly, there is a lot to think about when it comes to analytics and IT. The good news is that C-level executives are seeing the potential and the benefits. In talking about the digital tracking and analysis of trucking logistics, Mike Maris, senior director of transportation and logistics at Motorola Solutions, recently said, "Companies that rely on excellent logistics, such as package carriers and telcos, hook up black-box mobile communications in trucks that feed information about where the truck is going and also how it is being operated. The information helps companies analyze why some drivers have better gas mileage than others, and also aids in being able to prescribe training in areas, such as trying to use the shift better or following the same route. One of our business partners reported that they had seen double-digit decreases in fuel costs since instituting such a program."
With gains like this, it isn’t going to take companies long to see the value of real-time analytics—and to commit the necessary budget to IT so it can get the job done.
See more on this topic by subscribing to Network Computing Pro Reports The Data Mastery Imperative (subscription required).