The Journey Along the AIOps Innovation Continuum
The innovation arc for AIOps now clearly extends from the cloud to the data center and all the way to the customer edge.
March 5, 2021
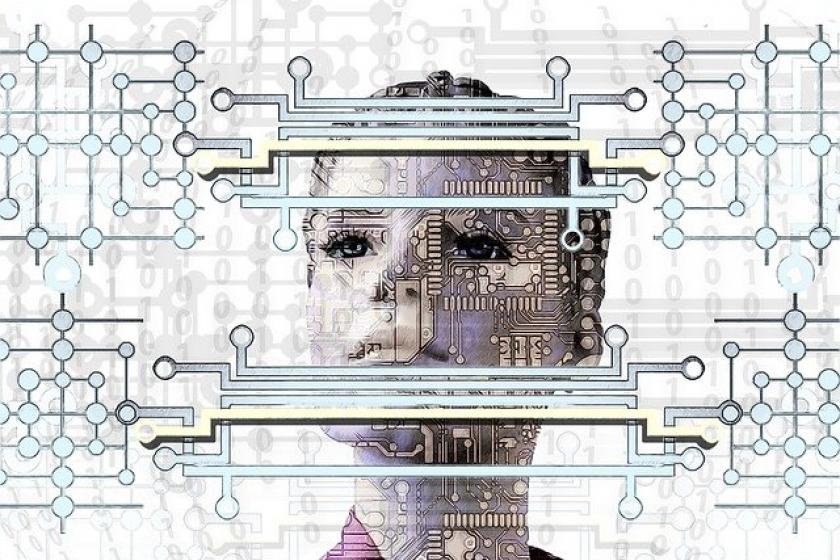
A recent IEEE survey of global CIOs and CTOs identified artificial intelligence (AI) and machine learning (ML) as the most important technologies in 2021. It’s not surprising that many in the industry consider AI and ML essential features of the technology landscape, able to harness the insights and power of the growing and endless deluge of data that flows from connected users and devices. One need look no further than AIOps – the application of AI to IT operational activities – as a case study in the application of these emerging technologies for improved enterprise networking. In fact, we are in the early stages of the AI revolution for networking. What began just a few years ago with applying AI to facilitate anomaly detection and troubleshooting in the data center has evolved to monitoring and predicting failure at the edge. Next, new insight-driven and outcome-based features across the entire network infrastructure are destined to transform business operations entirely by melding technical and business information together.
The AIOps continuum begins with solutions that typically address operational efficiency at the network core or data center. These AIOps features primarily analyze and predict network behavior—nothing more. The next step along the continuum, just coming into operation for early adopters, advances AIOps from IT systems monitoring to root cause analysis and problem identification and, in the most sophisticated instances, remediation. Taking AIOps to the next logical step sees AI and ML in use across infrastructure and application monitoring to proactively identify and potentially address issues autonomously before they even affect network performance or user experience.
Bolstering operational efficiency at the edge…
Next on the continuum? AIOps is being applied to monitor and autonomously correct issues at the network edge instead of only bolstering network resiliency at the network core, representing a significant inflection point along the continuum.
Failure at the WAN edge can be catastrophic for an enterprise site, causing hours of network downtime, hurting the customer experience, and preventing revenue-generating activity. AIOps that target WAN edge systems—such as routers, SD-WAN devices, and firewalls—optimize data flow across the edge, predict network traffic patterns across the complete route, and identify and triage possible single-point-of-failure edge devices. This allows the network to heal itself, edge-to-edge, promoting greater operational efficiency and protecting business continuity.
…and across the network
Extending AIOps to the edge also increases the data pool for ML models to continuously learn and optimize the use of AI across the network. What’s more, as the number of users, devices, and applications on enterprise networks grow, the volume of available data on associated WANs also skyrockets. That means ML models can simultaneously absorb, contextualize and analyze data from locations throughout a distributed network to assess the impact on end-users, both within the individual network and across the entire WAN population. AI can then assess overall network status, identify anomalous situations, automatically open service tickets, and alert as well as provide triage recommendations to service agents and engineers.
As the performance baseline builds over time through round-the-clock ML analyses, the system increases its ability to recognize unique operational characteristics and identify service-disrupting symptoms faster and more accurately, which improves the resolution of undesirable network behavior. Even when AIOps features at the edge do not autonomously correct problems, they function as an early warning system, often providing diagnoses of potential hardware failure or chronic site issues. This enables early action that can completely preempt any catastrophic disruption.
Insight-driven information services
Today, AIOps capabilities are being used across IT operations to monitor, triage, and even preempt network issues at the core and across the WAN edge. Next along the AIOps journey, Managed Services Providers (MSPs) are expanding AIOps applications more broadly across the digital enterprise environment. The opportunity lies in uncovering data-driven insights that enable the enterprise to deploy changes or corrective actions to benefit other parts of the business.
For example, a retailer may use in-store kiosks to help shoppers find product information or checkout. Any glitch in that kiosk system threatens to upset the customer experience and affect business. Digital experience monitoring (DEM) can detect disruption to a kiosk or kiosk system, while AIOps pinpoints the potential problem – for instance, classifying the issue as a likely point-of-sale (POS) system failure or a problem with the kiosk display screen. The MSP may not have direct access to system screens or the POS platform but can arm the customer with insights from AIOps that better inform the overall business. The customer then gains critical context for troubleshooting and remediation efforts across an increasingly complex network of devices, applications, and tools.
Business transformation through outcome-based services
But that is not the extent of AIOps possibilities. The next stage along the continuum will be truly transformative – moving beyond network and operational performance improvements to influence business decision-making.
An example can be found in the retail petroleum industry, where owner-operators depend on AI and ML data to monitor and even validate fuel levels in their tanks. A retailer’s network can collect and analyze data from the variety of systems installed on-site, including fuel levels from storage tank sensors; customer traffic patterns; and customer-based POS intelligence (how much, how often, and type of gas purchased). This on-site data can be synthesized with AIOps to corroborate inventory or, based on preset “rules” or policies, signal an issue with tank levels such as leaks or pilfering.
This is just one more mile marker along the AIOps innovation continuum.
Path to adoption
AI and AIOps present both an exciting opportunity for enterprises and an intriguing challenge for MSPs. We are at the very start of a complex journey toward much more efficient services, new capabilities, and true outcome-based services. The innovation arc for AIOps now clearly extends from the cloud to the data center and all the way to the customer edge. We see this in our efforts at Hughes, as well, where the AIOps innovation started out in our datacenter, moved to the cloud, and in the near term, will co-exist in the cloud and customer edge. The technology is just starting to mature – and there are opportunities on the horizon, including the realization of a ‘self-healing network.’ But this new reality also requires a new level of trust and cooperation.
With more business data exposed, new data partners, and new AI platforms, businesses and service providers face complex issues around privacy, security, data integrity, and business confidential insights and transactions. AIOps implementations can help build the infrastructure, the confidence, and the trusted team to tackle enterprise digital transformations leveraging AI and ML, the most important technologies of 2021.
Dan Rasmussen is SVP, North America Enterprise Division at Hughes Network Systems.