Build The Infrastructure For Big Data's 3 Stages
Learn the essentials to construct a shared-service pipeline that leverages a common set of infrastructure resources while still offering flexibility.
December 17, 2015
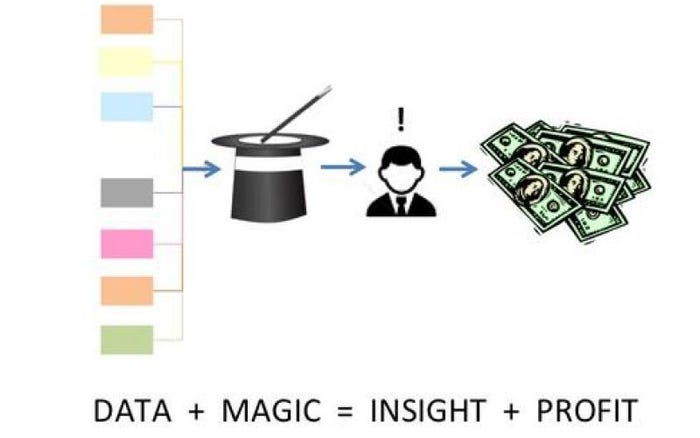
Much of the narrative around big data and analytics focuses on the "magic" that happens when sufficiently sophisticated software heuristics are applied to sufficiently large and useful datasets. Some writing on the subject almost gives the impression that you just have to wave a wand over your data and say "Hadoop!" to get insights that will transform your business.
But software doesn't happen without hardware. And not all datasets or analytic techniques are created equal. So for companies to successfully apply big data analytics to diverse business challenges across the enterprise, they need infrastructure that can:
Perform capacity intensive operations at sufficient speed to meet end user needs, and
Support a diversity of use cases with significantly varying workload profiles.
Oh, and one more thing: IT has to build the infrastructure for an analytics pipeline without breaking the bank.
An enterprise-level strategy for big data infrastructure is especially important because analytic initiatives are often very fragmented. Marketing embarks on a project here; compliance embarks on another one there. If each of these groups build their own analytic environments, there's bound to be a lot of redundant spending -- and a lot of redundant performance/capacity problem solving.
That's why it typically makes sense to construct a shared-service pipeline that leverages a common set of infrastructure resources across the business while still providing the flexibility to address analytic workloads with highly variable characteristics.